Member-only story
Counterfactuals and Causal Inference: A Comprehensive Guide

Arthur Mason
·10.4k Followers· Follow Published in Counterfactuals And Causal Inference: Methods And Principles For Social Research (Analytical Methods For Social Research)
7 min read 1.2k View Claps
97 Respond
<meta name="viewport" content="width=device-width, initial-scale=1.0"> <meta name="description" content="This article provides a comprehensive overview of counterfactuals and causal inference, including the key concepts, methods, and applications."> Counterfactuals and causal inference are two closely related concepts that are essential for understanding the relationship between cause and effect. A counterfactual is a statement about what would have happened if something else had happened. For example, we might say that if we had not taken that job, we would be rich today. Causal inference is the process of drawing s about cause and effect from data. In this article, we will provide a comprehensive overview of counterfactuals and causal inference. We will discuss the key concepts, methods, and applications of causal inference. We will also provide some tips on how to avoid common pitfalls in causal inference. <h2>Key Concepts</h2> **Counterfactuals** A counterfactual is a statement about what would have happened if something else had happened. Counterfactuals are often used to think about the past or to imagine the future. For example, we might think about what would have happened if we had won the lottery or if we had married someone else. Counterfactuals are essential for causal inference. This is because causal inference is based on the idea that we can compare what happened to what would have happened if something else had happened. **Causal Effects** A causal effect is the effect of one variable on another variable. For example, the effect of taking a drug on a person's health is a causal effect. Causal effects can be positive or negative. For example, taking a drug might improve a person's health or it might make it worse. **Potential Outcomes** Potential outcomes are the possible outcomes that could have occurred in a given situation. For example, if we flip a coin, there are two potential outcomes: heads or tails. Potential outcomes are essential for causal inference. This is because causal effects are defined as the difference between potential outcomes. **Treatment Effects** A treatment effect is the effect of a treatment on a group of people. For example, the effect of a new drug on a group of patients is a treatment effect. Treatment effects can be estimated using a variety of methods, including randomized experiments, observational studies, and propensity score matching. <h2>Methods of Causal Inference</h2> There are a variety of methods that can be used to make causal inferences. The most common methods are: **Randomized experiments** Randomized experiments are the gold standard for causal inference. In a randomized experiment, participants are randomly assigned to different treatment groups. This ensures that the treatment groups are comparable on all other factors, so that any differences between the groups can be attributed to the treatment. **Observational studies** Observational studies are studies in which participants are not randomly assigned to treatment groups. Instead, participants are observed and their outcomes are compared. Observational studies are often used to study the effects of treatments that cannot be randomized, such as the effects of smoking or exposure to air pollution. **Propensity score matching** Propensity score matching is a statistical method that can be used to estimate treatment effects from observational data. Propensity score matching creates a comparison group that is similar to the treatment group on all other factors, so that any differences between the groups can be attributed to the treatment. **Regression discontinuity design** Regression discontinuity design is a statistical method that can be used to estimate treatment effects from observational data. Regression discontinuity design uses a discontinuity in a variable to create a comparison group that is similar to the treatment group on all other factors. **Instrumental variables** Instrumental variables are variables that are related to the treatment but not to the outcome. Instrumental variables can be used to estimate treatment effects from observational data. <h2>Applications of Causal Inference</h2> Causal inference has a wide range of applications in social science research. Some of the most common applications include: **Evaluating the effectiveness of social programs** Causal inference can be used to evaluate the effectiveness of social programs, such as job training programs or educational interventions. By comparing the outcomes of participants in the program to the outcomes of a comparison group, researchers can estimate the causal effect of the program. **Studying the effects of public policy** Causal inference can be used to study the effects of public policy, such as the effects of a new tax law or a new environmental regulation. By comparing the outcomes of people who are affected by the policy to the outcomes of people who are not affected by the policy, researchers can estimate the causal effect of the policy. **Assessing the risk of health outcomes** Causal inference can be used to assess the risk of health outcomes, such as the risk of heart disease or cancer. By comparing the outcomes of people who are exposed to a risk factor to the outcomes of people who are not exposed to the risk factor, researchers can estimate the causal effect of the risk factor. <h2>Tips for Avoiding Common Pitfalls in Causal Inference</h2> There are a number of common pitfalls that can occur when conducting causal inference. Some of the most common pitfalls include: **Selection bias** Selection bias occurs when the participants in a study are not representative of the population that is being studied. This can bias the results of the study and lead to incorrect s. **Confounding** Confounding occurs when a third variable affects both the treatment and the outcome. This can bias the results of the study and lead to incorrect s. **Instrumental variable bias** Instrumental variable bias occurs when the instrumental variable is not actually related to the treatment. This can bias the results of the study and lead to incorrect s. Counterfactuals and causal inference are two powerful tools for understanding the relationship between cause and effect. By using these tools, researchers can gain insights into the effects of social programs, public policy, and health interventions. However, it is important to be aware of the common pitfalls that can occur when conducting causal inference. By avoiding these pitfalls, researchers can ensure that their studies produce valid and reliable results.
Counterfactuals and Causal Inference: Methods and Principles for Social Research (Analytical Methods for Social Research)
by Stephen L. Morgan
4.6 out of 5
Language | : | English |
File size | : | 5239 KB |
Text-to-Speech | : | Enabled |
Screen Reader | : | Supported |
Enhanced typesetting | : | Enabled |
Word Wise | : | Enabled |
Print length | : | 526 pages |
Create an account to read the full story.
The author made this story available to Nick Sucre members only.
If you’re new to Nick Sucre, create a new account to read this story on us.
Already have an account? Sign in
1.2k View Claps
97 Respond
Join to Community
Do you want to contribute by writing guest posts on this blog?
Please contact us and send us a resume of previous articles that you have written.
Resources
Fiction
Non Fiction
Romance
Mystery
Thriller
SciFi
Fantasy
Horror
Biography
Selfhelp
Business
History
Classics
Poetry
Childrens
Young Adult
Educational
Cooking
Travel
Lifestyle
Spirituality
Health
Fitness
Technology
Science
Arts
Crafts
DIY
Gardening
Petcare
David E Stuart
Sarah Zettel
Yossi Ghinsberg
Helen Clarke
Lucas Bessire
Paula Yoo
Patrick Mcginty
Dave Pine
Shantel Silbernagel
Fred H Croom
Bex Gunn
Ted Kaczynski
Joshua Becker
Larry Baush
Mary Griffith
Zigzag English
Susan M Orsillo
Gary Dean Quesenberry
Joann Cianciulli
Robert Axelrod
William F Keegan
Jared Derksen
Irene Mceachen
Mark Booth
Simon Pridmore
Steve Schwartz
Cj Andersen
Richard Holmes
Wendy Doniger
Bill Hammack
Bill Gutman
Billy Griffiths
Manoj Sharma
James Dashner
Rachna Chhachhi
Herschel Knapp
Blake Sebring
Linda Bauer
Kasun Indrasiri
Curvebreakers
Claudia Mazzucco
Kristin N Spencer
Krista Tippett
James Patterson
Frank S Ring
Jackie Bolen
Ramona Finn
Jeff Wheeler
Bharath Ramsundar
Leah Day
David Benjamin
Paul Brummell
Zecharia Sitchin
Charles Duhigg
Bryan Irwin
Vernon G Zunker
Phoebe Bailey
The Uk Mathematics Trust
Laurie Rubin
Jelena Bogdanovic
Ryan Johnston
John Brierley
Jitendra Chouksey
Nrup Parikh
Matthew Bowling
Sang H Kim
Erin Mckittrick
Gianna Sobol
Kristopher Martel
Brian Crist
Mark Young
Charlotte E English
G William Barnard
Pat Drake
Joan Roughgarden
Colleen Graves
Supersummary
Matt Doeden
Ross Edgley
Elizabeth Thompson
Tom Dodd
Bill Karwin
T Whitmore
Brandon Sanderson
Tony Ortega
Christopher Banecks
Heather Long
David E Johnson
Phil Genova
Gail Fay
Erin Beaty
Sheila Mackechnie Murtha
Rob Casey
Blaine Bartel
Michael Hartman
Cheryl Marlene
Phil Robertson
Marc Bona
Daniel J Velleman
Enzo Tonti
Rabbi Jason Sobel
Issai Chozanshi
Bill Moeller
Edwin H Friedman
Kent Hrbek
James R Payne
Lisa Dorfman
Curt Sampson
Oscar Nilson
Chris Fischer
Steve Biddulph
Sara Low
Dina Nayeri
Farah Heron
Evan Purcell
Bill Nowlin
Dick Edie
Bryan Mann
Bill Gladstone
Vanessa Lapointe
Jacques Devore
Alan Lawrence Sitomer
Jamie Foxx
Leland Chant
Mike Westerfield
Yau Ming Ng Thompson
Erin Mcrae
Virginia Smith Harvey
Ross Bonander
Susan Shelby Torrance
Dean Keith Simonton
Jennifer Shannon
Brad States
Geraldine Van Bueren
Rob Steger
Sam Harris
Marsha Vanwynsberghe
Craig Chappelow
Max Youngquist
Max Lugavere
Melissa Layne
John C Norcross
Gary Player
Bob Glover
Louis Sachar
Gavin Weightman
Ian Tuhovsky
Megan Don
Luciano Floridi
Thomas Bailey
Jon M Sweeney
Eric A Weiss Md
Tiffany Bergin
Bill Schneider
Robert Bruce Thompson
Zoe Hana Mikuta
Davi Kopenawa
Special Tactics
Simon Baron Cohen
Frank Giampaolo
Martin Davies
Sandi Mann
Elizabeth A Stanley
Maggi Savin Baden
Izzy Judd
Fodor S Travel Guides
Cherie Dimaline
John Mccannon
Dan Yaccarino
Claire Russell
Carlos Castaneda
Shawn Levy
Victoria Johnson
Tony Guerra
Mark Solms
Jakub Marian
Doug Scott
Felicity Aston
Bjorn Kiggen
Kiera Cass
Jake Jacobson
Steven M Levy
Mike Gibson
Rachael Scdoris
Clement Salvadori
John Mccollister
Lee Gutkind
Tina Cassidy
Dawn Huebner
Mark Vanhoenacker
Jackie Brown
Megan Mcgrory Massaro
Suzannah Rowntree
Bob Duff
Linda D Dahl
Ofer Gal
Pedro Urvi
J D Gauchat
Diana Wynne Jones
Ron Elbe
Dashka Slater
Bev Pettersen
Katie Singer
H P Lovecraft
Zavonda Vinson Parrish
Donncha Hanna
Sanford Holst
Ingrid Chalufour
Stephen L Morgan
Diondre Mompoint
Richard Post
Nancy Romita
Jarrett Dapier
Hilary Nangle
T Edward Nickens
Harlan Coben
Rick Reilly
Stephan A Hoeller
Stephen Arterburn
Bill Boyum
John H Holland
Isabel Fonseca
Jen Castleberry
Paul Halpern
Sue Enquist
Genius Reads
J Robert King
Douglas W Ota
Editors Of Garden And Gun
Beck Weathers
Hayley Mitchell Haugen
Tom Humphrey
Scott Wilson
Michael D Alessio
Robert F Burgess
Morgan Oostra
Elliot Kay
Jamie Aten
Thad Beery
Peter Jackson
Pete Spencer
Kat Kruger
Dawn Hadley
Editors Of Sports Illustrated
Bob Duchesne
Jessica Taylor
Yakima Canutt
Martyn Denscombe
Dr Julissa Hernandez Nd Cnhp
Michael J Epstein
Michael Lempert
Freddie Fernandez
Richard B Pelzer
Karen Palacios Jansen
Bode Miller
Jedd K Parkinson
Gregg Jackson
Robert A Pelcovits
Frederica Relly
P J E Peebles
Justin Lichter
Laurence Price
Sandra Davidson
Peter Aitken
Marilee Lebon
Tim Weston
Gary Nicol
Doug Fletcher
Simon Buxton
Paris Williams
Dan Blanchard
Kate Darling
Daniele Benedettelli
Holly Donahue Singh
Marisa Imon
Callum Roberts
Carson Sievert
D C Haenlien
Ken Venturi
Lynette Rushton
Rod Powers
Christian Smith
Chris Ferrie
Neil D Jespersen
General
Deborah Blum
Sabaa Tahir
Sandra Berenbaum
Marty Gitlin
Jon Loeliger
Matt Parker
Billy Martin
Dan Murphy
Emma Griffin
Marie Viljoen
David Joyce
J Douglas Faires
Bill Miller
Helen Irlen
Andrea Cremer
Betsy Herman
Dr Nancy L Nolan
Rupert Spira
Kasey Edwards
Herbert Dorsey
Tara Bianca
Jonathan Crichton
Dan Garner
Emma Cannon
Paul Bellow
Ernest Raymond
Henry M Cowles
Bill Bennett
Jacques Steinberg
Ta Nehisi Coates
Kevin Sverduk
Jeremy Paxman
Peter Julius Sloan
Olivia Gordon
Michael Tlanusta Garrett
Wanza Leftwich
Holger Schutkowski
Karen Armstrong
David Price
Elizabeth King
Kenny Dill
Laura Nowlin
Paul Francis
Rachel Burgess
Jamie Dumas
J T Williams
Chris Sims
Silvia Dunn
Jacqueline B Persons
Marion Zimmer Bradley
Mindy Mcginnis
Richard W Fisher
Eric H Cline
James W Finegan
Michael W Eysenck
Shannon Sovndal
Nicole R Taylor
Henry Charles Lea
Jeffrey Lindsey
Joseph Campbell
Mike Veny
Dylan Tomine
Conway X Bowman
Terry Pratchett
James P Allen
George C Thomas
Rocky Mcelveen
Ellen Schuthof Lesmeister
Sophia Freeman
Melissa Abramovitz
Gary Kamiya
Carl B Tolman
Robert Greene
Hugh Neill
Adiba Jaigirdar
Dinah Bucholz
Jim Greenwood
James Alexander Currie
Joe Byers
Shelby Mahurin
David Halberstam
Deborah J Rumsey
Janice Selekman
Michael Sullivan
Joy Hakim
David Nirenberg
Robert Byron
Sharon Bergen
Manly P Hall
Zane Grey
Greg Witt
Charles Goodwill
Holly Jackson
John Muir Laws
R E S
Zach Schonbrun
C D Holmes Miller
Jessica Denay
Chris Napier
Clifford A Pickover
Mosby
Kate Marchant
Ginger Sinsabaugh
Dan Hamilton
Jennifer Kolari
Meagan Trayler
Mark Lehner
Mercedes Lackey
George Mahood
Tiffany Loggins Psyd
Charles Simpson
Al Walsh
Sue L Hamilton
Russ Harris
Sara Dyer
John Kettle
Joie Jager Hyman
Jojo Siwa
Bill Streever
Ezekiel Eversand
Stephanie Manley
Marie Max House
Marcus Brotherton
Stanislas Dehaene
Bill Patton
Joshua Foer
Elizabeth Winthrop
Gwendoline Smith
S W Wilcox
Charlie Craven
Paul Levy
Henry Nicholls
Anya Kamenetz
Wendy Hinman
R E Skibiski
Thomas Cleary
Jane Nelsen
Elsevier
Sheridan Anderson
Max Help Workbooks
Joseph Edminister
Rough Guides
Rick Steves
Margo Armstrong
Sharmila Desai
Fiona Danks
Carolyn Schulz
Kindle Edition
Robert P Beebe
Kevin Marx
Michael J Tougias
Aylette Jenness
Rebekah Nathan
Scarlett Curtis
Jessica Wiebe
Jane Bottomley
Colin Thubron
Ian Wilson
Steven Hassan
Charles A Rhodus
Vukota Boljanovic
Carlos Torres
Karyn D Hall
Valeria Ray
Kevin C Kelleher Md Md
Richard Rohr
Michael Matthews
Stacie Mahoe
Styrling Strother
Michael Volkmar
Chris Sajnog
Brienne Murk
Pat Cohen
Light bulbAdvertise smarter! Our strategic ad space ensures maximum exposure. Reserve your spot today!
Good Author
- Gene PowellFollow ·6.8k
- Allen ParkerFollow ·4.5k
- Billy PetersonFollow ·6.3k
- Howard PowellFollow ·8.3k
- Ron BlairFollow ·9.6k
- Dakota PowellFollow ·19.6k
- Ivan TurgenevFollow ·11.1k
- Dan HendersonFollow ·15.3k
Recommended from Nick Sucre
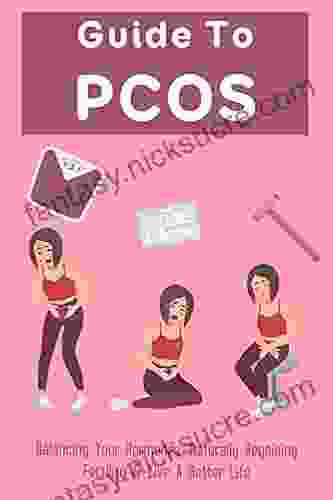

Balancing Your Hormones Naturally: Regaining Fertility...
Hormones play a vital role in our...
·4 min read
1.4k View Claps
90 Respond
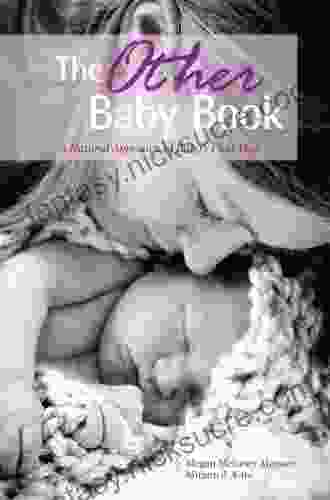

The Other Baby Book: A Comprehensive Guide to Baby's...
The Other Baby...
·4 min read
1.5k View Claps
90 Respond
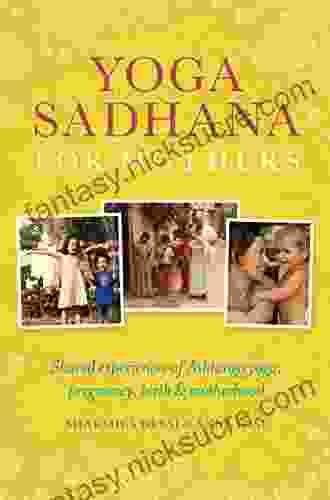

A Comprehensive Guide to Yoga Sadhana for Mothers:...
Motherhood is a...
·6 min read
27 View Claps
5 Respond
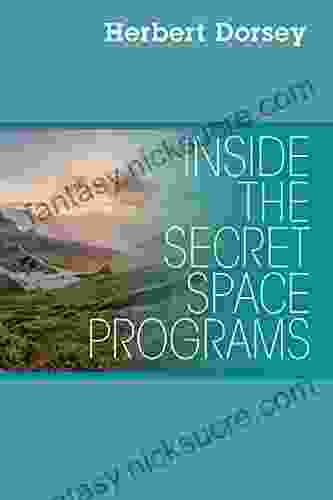

Inside the Secret Space Programs
An Exposé...
·6 min read
492 View Claps
48 Respond
The book was found!
Counterfactuals and Causal Inference: Methods and Principles for Social Research (Analytical Methods for Social Research)
by Stephen L. Morgan
4.6 out of 5
Language | : | English |
File size | : | 5239 KB |
Text-to-Speech | : | Enabled |
Screen Reader | : | Supported |
Enhanced typesetting | : | Enabled |
Word Wise | : | Enabled |
Print length | : | 526 pages |